The need for data engineers is skyrocketing, and consequently, many people are turning toward data engineering for their careers. Although the job offers good money and comfort, it’s essential to get an idea of the amount of stress it involves. Since some people can handle more stress than others, it can become a deciding factor for many.
Data engineering can be a stressful job with many tools and techniques to choose from. Deadlines and work pressure are also there. And apart from that, the communication gap between data engineers and non-tech managers, lack of meaning, and boredom can also lead to frustration.
In this article, we will discuss the job responsibilities of data engineers and the work pressure they face at the office. We’ll also look at how a sense of meaning and fun projects can help data engineers enjoy their job.
Important Sidenote: We interviewed numerous data science professionals (data scientists, hiring managers, recruiters – you name it) and identified 6 proven steps to follow for becoming a data scientist. Read my article: ‘6 Proven Steps To Becoming a Data Scientist [Complete Guide] for in-depth findings and recommendations! – This is perhaps the most comprehensive article on the subject you will find on the internet!
Table of Contents
Stress in Data Engineering Depends on the Company?
Keep in mind that these things are subject to one’s own experience. In this article, I’ve given some general pointers about what is happening in the industry as of writing. Based on your experience as a data engineer, you may disagree with what is explained here.
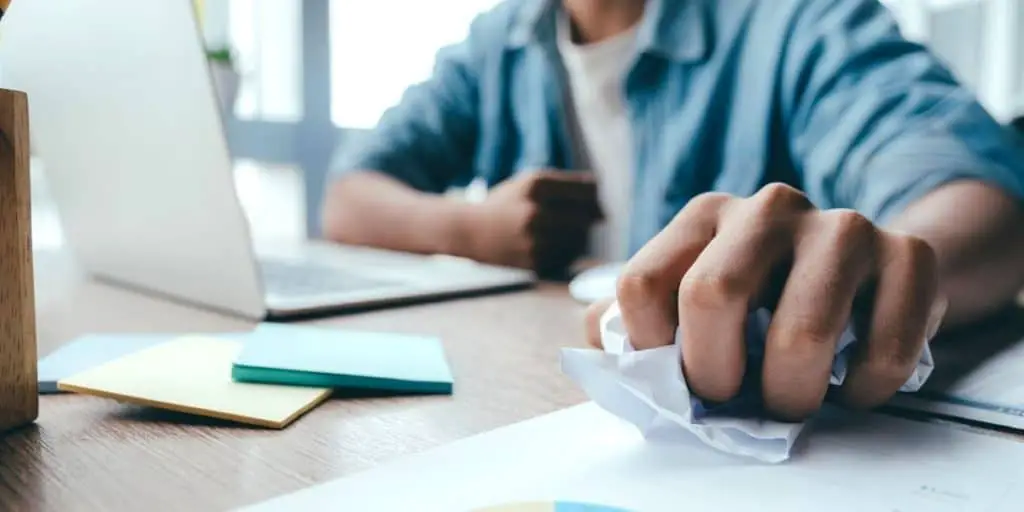
Data engineers usually work around 40 hours a week, though they may have to work more. The day-to-day activities of a data engineer include creating data pipelines and applying machine learning algorithms to them. They also compile and process data to make it suitable for analysis.
To answer the question, whether data engineering is stressful depends mostly on the company you’re working in. It would be incorrect to put a blanket statement like “data engineering is very stressful.” It’ll lead people to form misconceptions regarding the field.
Data engineering as such is not stressful; in fact, it can be quite fun. You get to find creative ways of processing data, write code for automating the boring stuff, and learn about the latest technology to have the edge over other data engineers.
So how does your place of work affect job stress? Well, tech giants and big banks hire many programmers and data engineers. But most data engineers consider these jobs to be soul-sapping and stressful. Although they pay well, data engineers try to work at places where they can find more fulfillment from their job.
One reason for this is the competition-induced work pressure and deadlines at such big companies. Another reason is that whatever hard work you’re putting in is ultimately going toward making rich people even richer. It often doesn’t benefit society in any way.
Giant corporations usually have a culture of grind. They don’t care much about employee health and happiness. Amazon and Netflix are examples of places where you may earn good money, but you will be sucked dry if you’re not used to handling all the stress and pressure. On the other hand, smaller companies and startups don’t force you to hustle so much.
So if you’re concerned about working at a stressful place where you end up hating your job, you should carefully choose what kind of company you want to work for. How stressful your position can be entirely company-specific.
I’ve discussed this in detail in another article on whether data engineering is boring. You can read it here: Is Data Engineering Boring? Or Is It a Fun Career?
Why Do Data Science Professionals Switch Their Jobs?
Data engineers solve many problems on the computer every day. However, apart from what they face on the screen, data engineers also have a few other challenges at work. These are things that can further increase your stress and make the job more intense.
All four of the following reasons have been verified to exist. Data scientists and data engineers have left their jobs because of one of these reasons. It’s essential to understand them before you begin working as a data engineer. As such, this section can also be titled “be prepared for the following when jumping into data engineering.”
Here is why data scientists, data analysts, and data engineers are leaving their jobs:
Technical Requirements
Data engineering requires a lot of technical knowledge. You need to be a strong coder before you can become a data engineer. There are many tools and techniques to choose from, and picking the right tool for a particular problem is a big part of the job. Although you don’t need to know everything, the more you know, the more you grow.
You also need to keep up with everything and keep growing continuously to stay in the game. This can be a source of stress and burden for some people, while it may prove to be mentally stimulating and exciting for others.
Communication Gap
Data science can be a confusing field. Sometimes even people working in the industry don’t know the exact differences between data scientists, data engineers, and data analysts. As such, non-technical managers don’t understand how data science works, leading them to misinterpret their work.
A lot of the time, data science professionals are not provided the right questions to answer. Companies fail to get the most out of their data due to this communication gap. Data engineers often find it very challenging to explain the complexity of data science problems. This often leads to frustration and misinterpretation of various kinds.
Uncertainty
When a software engineer builds a piece of software, it is sure to work as intended. However, that’s not the case with data scientists and data engineers. It’s very much possible to get a different result every time you run the same data science model. It cannot be compared to a print statement, which outputs the same result every time.
Data science is not like other fields. When it comes to data science, you don’t know how big or small results you’ll get. In fact, you never know if you’ll get any significant results at all. A person may be doing everything right, but the solution or output still may not work.
However, I must mention that in the case of data engineering, the uncertainty is much less than in the case of data science. This is because the machine learning models that data engineers implement into the data pipeline have already been verified and tested at some level.
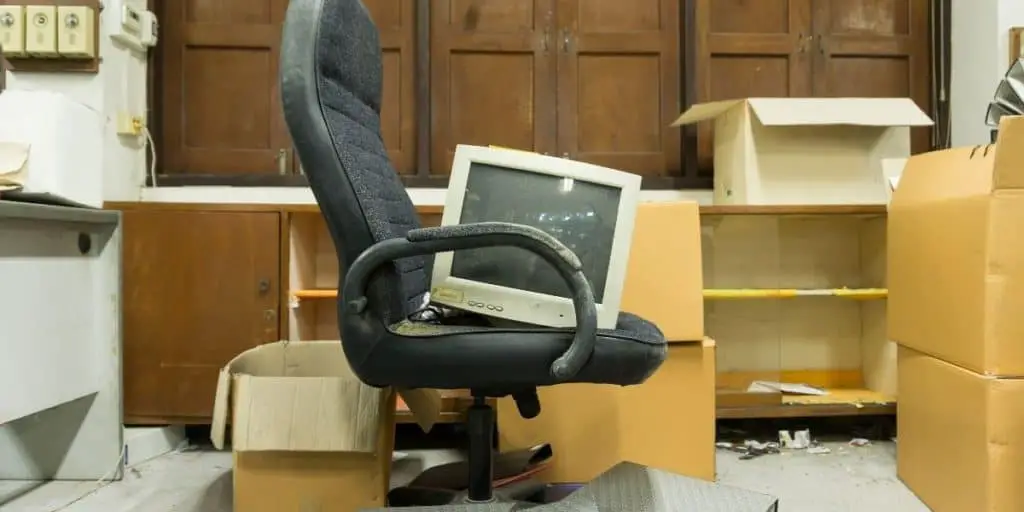
Infrastructure
Data scientists, data analysts, and data engineers don’t always have the most optimal working conditions. We’ve already discussed that data engineers’ work involves data scientists and data analytics in smaller companies. And sometimes, these organizations lack suitable infrastructure.
If you don’t have reliable infrastructure, it becomes tough to effectively process, analyze, and solve data-related problems. Data science professionals have been found to leave their jobs for this reason as well.
By the way, you also need infrastructure even to perform research on your own. This means it will cost you quite a bit to set up a suitable infrastructure for learning, experimenting, and researching.
Author’s Recommendations: Top Data Science Resources To Consider
Before concluding this article, I wanted to share few top data science resources that I have personally vetted for you. I am confident that you can greatly benefit in your data science journey by considering one or more of these resources.
- DataCamp: If you are a beginner focused towards building the foundational skills in data science, there is no better platform than DataCamp. Under one membership umbrella, DataCamp gives you access to 335+ data science courses. There is absolutely no other platform that comes anywhere close to this. Hence, if building foundational data science skills is your goal: Click Here to Sign Up For DataCamp Today!
- IBM Data Science Professional Certificate: If you are looking for a data science credential that has strong industry recognition but does not involve too heavy of an effort: Click Here To Enroll Into The IBM Data Science Professional Certificate Program Today! (To learn more: Check out my full review of this certificate program here)
- MITx MicroMasters Program in Data Science: If you are at a more advanced stage in your data science journey and looking to take your skills to the next level, there is no Non-Degree program better than MIT MicroMasters. Click Here To Enroll Into The MIT MicroMasters Program Today! (To learn more: Check out my full review of the MIT MicroMasters program here)
- Roadmap To Becoming a Data Scientist: If you have decided to become a data science professional but not fully sure how to get started: read my article – 6 Proven Ways To Becoming a Data Scientist. In this article, I share my findings from interviewing 100+ data science professionals at top companies (including – Google, Meta, Amazon, etc.) and give you a full roadmap to becoming a data scientist.
Conclusion
Like every other job on the planet, data engineering has pros and cons. Deadlines and normal work pressure are to be expected. But it can be incredibly stressful if you’re working in a competitive environment for a big company.
On the contrary, if you’re in an organization that values employee health and happiness, you will be much better off. If you work at a place aiming to make the world a better place, a sense of purpose and meaning will be added to your work. Then, even if the job is intense, you’ll enjoy it and fulfill your responsibilities enthusiastically.
BEFORE YOU GO: Don’t forget to check out my latest article – 6 Proven Steps To Becoming a Data Scientist [Complete Guide]. We interviewed numerous data science professionals (data scientists, hiring managers, recruiters – you name it) and created this comprehensive guide to help you land that perfect data science job.
Affiliate Disclosure: We participate in several affiliate programs and may be compensated if you make a purchase using our referral link, at no additional cost to you. You can, however, trust the integrity of our recommendation. Affiliate programs exist even for products that we are not recommending. We only choose to recommend you the products that we actually believe in.
Recent Posts
Data science has been a buzzword in recent years, and with the rapid advancements in artificial intelligence (AI) technologies, many wonder if data science as a field will be replaced by AI. As you...
In the world of technology, there's always something new and exciting grabbing our attention. Data science and analytics, in particular, have exploded onto the scene, with many professionals flocking...