Data science has been a buzzword in recent years, and with the rapid advancements in artificial intelligence (AI) technologies, many wonder if data science as a field will be replaced by AI. As you begin to explore this topic, it’s important to understand the key differences between data science and AI, as well as how they can complement each other.
No, data science won’t be completely replaced by AI. AI indeed will automate and enhance certain aspects of data science, but human intervention and creativity will remain essential. While AI tools can help analyze large volumes of data, human expertise is still needed to truly interpret that data and extract valuable insights.
In this article, you can expect to learn more about the growing relationship between data science and AI, specifically how AI can aid data scientists in their work, and the areas where human skills will continue to prevail. Ultimately, this article will demonstrate how AI and data science work hand-in-hand, rather than one replacing the other.
Important Sidenote: We interviewed numerous data science professionals (data scientists, hiring managers, recruiters – you name it) and identified 6 proven steps to follow for becoming a data scientist. Read my article: ‘6 Proven Steps To Becoming a Data Scientist [Complete Guide] for in-depth findings and recommendations! – This is perhaps the most comprehensive article on the subject you will find on the internet!
Table of Contents
The Automation Process in Data Science
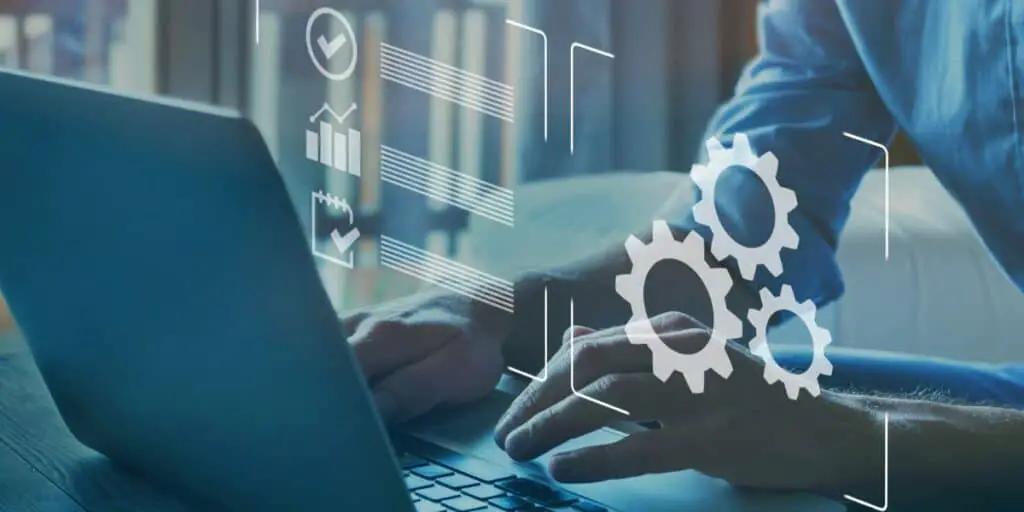
Emerging AI Tools for Automation
As a data scientist, you may have noticed the rise of automated AI tools that are making their way into the field of data science. These tools aim to simplify tasks like data preprocessing, model selection, and hyperparameter tuning, which can be time-consuming for you and other data science professionals. A few notable examples of these tools include:
- AutoML platforms, which can automatically choose the most suitable model for your dataset and even refine the model through optimization processes.
- Data cleaning tools, which can identify and handle missing or inconsistent data automatically, thus saving your time in data preprocessing.
- Feature engineering tools, such as Deep Feature Synthesis, that can automatically generate meaningful features from raw data for better model performance.
By staying informed about these emerging tools, you can leverage their potential to enhance your data science workflow.
Machine Learning and Deep Learning
Another key aspect of the automation process in data science revolves around the application of advanced machine learning and deep learning techniques. These techniques strive to improve the performance of predictive models by learning from data, without the need for explicit programming. Some examples include:
- Machine learning algorithms, such as decision trees, support vector machines, and clustering techniques, which can be used to analyze data and make predictions based on patterns they’ve learned.
- Deep learning frameworks, like TensorFlow and PyTorch, which are commonly used to build powerful neural networks and can tackle complex tasks like image recognition, natural language processing, and speech synthesis.
As a data scientist, expanding your knowledge of machine learning and deep learning can prepare you for the inevitable integration of these techniques in the automation of data science tasks.
To summarize, automation in data science is an emerging trend with the potential to make your work more efficient and effective. Stay updated on the latest AI tools for automation, and continue building your expertise in machine learning and deep learning. In doing so, you’ll be well-equipped to adapt to the changing landscape of your field.
Implications for Data Scientists
Changes in Roles and Skills
As automation and AI continue to advance, the field of data science will inevitably evolve. You, as a data scientist, will need to adapt and enhance your skillset to stay relevant in the industry. Some of the tasks you currently perform may be automated, but this also means new opportunities to focus on more complex and valuable tasks. For example:
- Shifting from manual coding to developing and refining AI algorithms.
- Moving from basic data analysis to extracting deeper, more meaningful insights.
- Expanding your expertise to interdisciplinary fields related to data science.
To keep up with these changes, it’s essential for you to continuously learn and stay updated with the latest industry trends. Some potential skills to hone include:
- Advanced machine learning and deep learning techniques.
- Data storytelling and visualization.
- Domain-specific expertise, such as finance or healthcare.
Impact on Job Market
The increasing use of AI and automation in data science might bring mixed outcomes for the job market. While certain roles might become redundant, the demand for skilled data scientists who can successfully leverage automation will continue to rise. Here’s what you may expect:
- Fewer job opportunities for entry-level data scientists, as simpler tasks may be automated.
- Increased demand for data scientists with specialized skills and domain knowledge.
- An emphasis on hiring professionals who can adapt to rapid technological advancements.
Employment opportunities for data scientists are not expected to diminish significantly, but it’s critical that you focus on building expertise in areas less likely to be automated, such as:
- Analyzing complex, unstructured data.
- Addressing ethical concerns and bias in algorithms.
- Deploying advanced AI systems that involve high levels of human-AI collaboration.
In summary, automation and AI will certainly impact the data science field, but staying agile and up-to-date with industry trends will help you navigate these changes and prosper in your career.
Potential Benefits of Automation in Data Science
Efficiency in Data Cleaning and Processing
Automation in data science can lead to significant improvements in the efficiency of data cleaning and processing. With the help of advanced technology and computers, machines can now autonomously:
- Identify and remove duplicate data entries
- Correct errors in data formatting and typing
- Fill in missing values based on patterns and relationships in the dataset
By automating these tasks, you can save valuable time and resources that would otherwise be spent on manual data cleaning. This allows you to focus on more important aspects of your work, such as problem-solving and decision-making.
Improved Analysis and Business Problem Solving
In addition to streamlining data cleaning processes, AI-powered automation can also enhance your ability to analyze data and solve business problems. Some potential benefits include:
- Faster processing: Computers can analyze vast amounts of data in a fraction of the time it would take a human, allowing you to draw insights and make decisions more quickly.
- Increased accuracy: Automated systems are less prone to human error, which means your analysis will be more reliable and precise.
- Enhanced pattern recognition: AI algorithms can identify patterns and relationships in data that might be difficult or impossible for humans to detect.
By leveraging these benefits, you can improve your overall approach to data science and enhance your ability to create value for your organization. Remember to stay up-to-date with advancements in technology, and always strive to sharpen your skills as a data scientist.
Limitations and Challenges in Automating Data Science
Creative and Complex Decision-Making
Although AI and automation are making strides in various fields, there are still limitations when it comes to automating data science completely. One notable challenge is handling creative and complex decision-making tasks. You see, data science often involves developing novel solutions for unique problems. While AI has demonstrated success in some areas, it often lacks the ability to approach problems with the same creativity and flexibility that the human brain provides. For example, when working with data in industries like finance, banking, and legal services, it becomes crucial to have a human touch for decisions that go beyond the scope of established algorithms.
Ethical Concerns and Bias
Another challenge in automating data science is dealing with ethical concerns and bias. AI models are trained on datasets, and these datasets can sometimes reflect the biases present in the real world. Consequently, it can unintentionally perpetuate these biases when making decisions. In fields such as HR and recruitment, this could lead to unfair treatment of candidates based on gender, race, or other factors. As a data scientist, addressing these concerns and promoting fairness requires:
- Actively identifying and mitigating biases in datasets and algorithms
- Ensuring transparency in the development and decision-making processes
- Collaborating with diverse teams to minimize potential blind spots
Moreover, the implementation of AI in various industries may raise ethical questions regarding data privacy, security, and human intervention. Balancing AI automation with ethical considerations will remain a significant challenge as data science continues to grow in influence.
To summarize, while AI automation is undoubtedly making an impact on parts of data science, fully replacing the human element is not yet feasible due to creative and complex decision-making, ethical concerns, and biases. For now, it’s crucial for data scientists and AI to work together in a collaborative approach to harness the strengths of both and overcome the challenges along the way.
Emergence of Generative AI and Its Impact
Generative AI has been evolving rapidly, leading to significant changes in various industries. It combines machine learning techniques with large volumes of data to generate entirely new content, pushing the boundaries of data science automation. One area where this technology is already having a notable impact is in the fields of media and marketing.
Applications in Media and Marketing
With the power of generative AI, creative processes in both media and marketing have become increasingly automated. Examples of generative AI applications in these fields include:
- ChatGPT: Developed by OpenAI, ChatGPT is an advanced language model that generates human-like text responses. It can assist you in drafting creative content, customer support communications, and even brainstorming ideas.
- Media synthesis: AI algorithms can now create original media, such as images and videos, mimicking the style or attributes of existing content. This capability opens up new possibilities for advertising campaigns and personalized content creation.
- ANQE: This technique, known as Adversarial Network Quantitative Evaluation, helps assess the quality and performance of generative models in marketing use cases, ensuring your AI-enhanced campaigns meet your goals.
The adoption of generative AI in media and marketing offers various benefits:
- Cost-effectiveness: Automated processes can reduce costs associated with hiring additional professionals or investing in costly editing tools.
- Increased productivity: By automating certain tasks, your team can focus on more complex, strategic responsibilities.
- Faster innovation: Generative AI can enable rapid idea generation and execution, leading to more innovative campaigns that resonate with your audience.
It’s important to remember, however, that while some tasks within media and marketing can be automated, it doesn’t mean these industries will be entirely replaced by AI. The human touch, creativity, and strategy are still essential to drive your campaigns and engage your audience.
In conclusion, generative AI is undeniably transformative, offering new opportunities for media and marketing professionals to leverage technology for creative purposes. As you continue to maintain the quality and innovation of your work, embracing AI can add value to your processes and help you stay ahead of the game.
Future Outlook of Data Science Automation
Shift in Occupations and Industries
As data science continues to evolve, the automation of certain tasks becomes inevitable. However, this does not mean that data scientists will be replaced entirely. Instead, it could lead to a shift in the focus of their jobs and the industries in which they operate.
- AI tools are increasingly being developed to enhance the capabilities of data scientists
- The future of data science will likely involve collaboration between humans and AI, rather than a direct competition for jobs
One potential outcome of this shift is the emergence of new job titles and descriptions tailored to the changing landscape of data science. As professionals adapt, they might find themselves working alongside AI-powered tools in areas such as:
- Natural language processing (NLP)
- Speech and video analytics
- Real-time data management
Collaboration Between Humans and AI
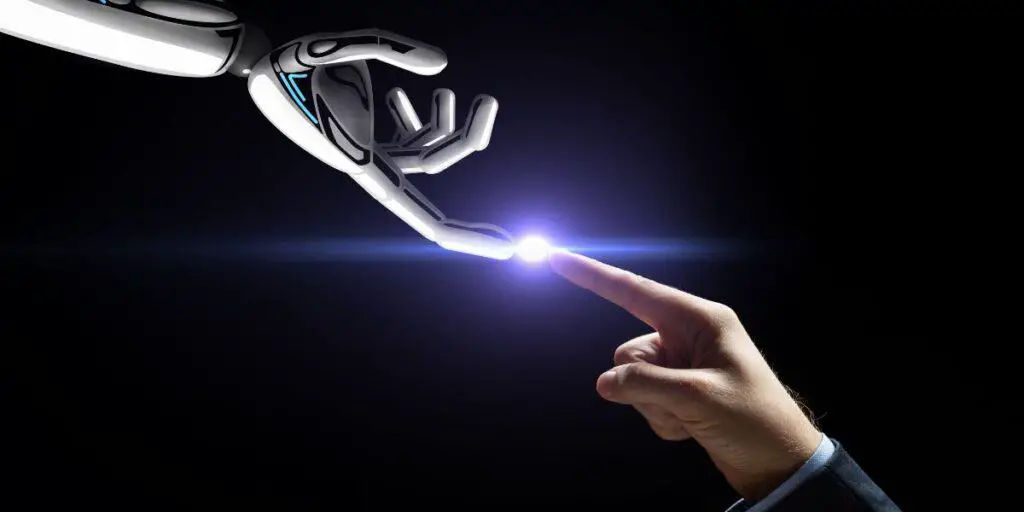
The key to success in this evolving field is to embrace the potential of AI tools while acknowledging the unique strengths that humans bring to the table. A few areas where human and AI collaboration could be effective include:
- Devising more strategic and targeted plans to tackle data-driven problems
- Enhancing the interpretation of complex data and identifying patterns that may otherwise go unnoticed
- Leveraging human intuition to make sense of AI-generated insights and guide decision-making
In order to make the most of this collaboration, you should:
- Keep up-to-date with the latest developments in AI and data science technologies
- Develop a strong foundation in both programming and traditional data analysis
- Embrace interdisciplinary approaches and be open to learning from other industries
By focusing on these areas, you can help to ensure a productive and harmonious relationship between humans and AI in the future of data science. Remember, the goal is not to see AI as a threat but rather as an ally in navigating the increasingly complex world of data.
Author’s Recommendations: Top Data Science Resources To Consider
Before concluding this article, I wanted to share few top data science resources that I have personally vetted for you. I am confident that you can greatly benefit in your data science journey by considering one or more of these resources.
- DataCamp: If you are a beginner focused towards building the foundational skills in data science, there is no better platform than DataCamp. Under one membership umbrella, DataCamp gives you access to 335+ data science courses. There is absolutely no other platform that comes anywhere close to this. Hence, if building foundational data science skills is your goal: Click Here to Sign Up For DataCamp Today!
- IBM Data Science Professional Certificate: If you are looking for a data science credential that has strong industry recognition but does not involve too heavy of an effort: Click Here To Enroll Into The IBM Data Science Professional Certificate Program Today! (To learn more: Check out my full review of this certificate program here)
- MITx MicroMasters Program in Data Science: If you are at a more advanced stage in your data science journey and looking to take your skills to the next level, there is no Non-Degree program better than MIT MicroMasters. Click Here To Enroll Into The MIT MicroMasters Program Today! (To learn more: Check out my full review of the MIT MicroMasters program here)
- Roadmap To Becoming a Data Scientist: If you have decided to become a data science professional but not fully sure how to get started: read my article – 6 Proven Ways To Becoming a Data Scientist. In this article, I share my findings from interviewing 100+ data science professionals at top companies (including – Google, Meta, Amazon, etc.) and give you a full roadmap to becoming a data scientist.
Frequently Asked Questions
Will AI affect data science jobs?
Yes, AI will impact data science jobs but not necessarily replace them. AI is likely to automate some tasks, making professionals more efficient and improving the field overall. Data scientists must adapt and embrace AI tools as part of their work.
Are data analysts being replaced by AI?
AI is not replacing data analysts entirely, but it may alter their roles. With AI handling routine tasks, data analysts will focus more on interpreting results, gaining insights, and providing strategic guidance to decision-makers.
What is the future of data science?
The future of data science is promising, with AI and machine learning being key drivers of growth. As businesses increasingly rely on data-driven decisions, the demand for skilled data scientists will continue to rise. In addition, new AI tools and techniques will likely enhance the capabilities and efficiency of data science professionals.
How will AI impact data engineers?
AI will likely change the way data engineers work but not eliminate their jobs. As AI tools improve automated data processing and management, data engineers will need to adapt and focus on higher-level tasks like designing robust systems and ensuring data quality.
Is automation taking over data science?
Automation is transforming data science by taking over some repetitive tasks, allowing professionals to focus on more complex and dynamic aspects of their work. However, automation is not taking over data science entirely, as human expertise remains crucial for interpreting results and providing contextual insights.
Will data science remain in demand in the future?
Data science is expected to remain in high demand in the future. As businesses and organizations continue to leverage data to gain insights, make informed decisions, and improve processes, the need for skilled data scientists will grow. Additionally, advancements in AI and automation will further boost the value and importance of data science in various industries.
BEFORE YOU GO: Don’t forget to check out my latest article – 6 Proven Steps To Becoming a Data Scientist [Complete Guide]. We interviewed numerous data science professionals (data scientists, hiring managers, recruiters – you name it) and created this comprehensive guide to help you land that perfect data science job.
Affiliate Disclosure: We participate in several affiliate programs and may be compensated if you make a purchase using our referral link, at no additional cost to you. You can, however, trust the integrity of our recommendation. Affiliate programs exist even for products that we are not recommending. We only choose to recommend you the products that we actually believe in.