Data science is a powerful tool that is useful across most industries. From technology to financial services, travel to agriculture, healthcare to retail, and everything in between, data science is essential. That is why this field is on so many students’ lists of careers to consider; however, is it easy to learn?
Data science is not easy to learn. Those who are best suited for this field need to be skilled in mathematics and have a natural affinity towards numbers and analytics. Still, with hard work, studying, and hands-on learning, students with different academic backgrounds can learn the needed skills.
There are specific personality traits that will make being a data scientist more enjoyable. On the same note, there are also tendencies to view and process data to help a data scientist experience more success and less frustration. Read on to explore these traits and tendencies further.
Important Sidenote: We interviewed numerous data science professionals (data scientists, hiring managers, recruiters – you name it) and identified 6 proven steps to follow for becoming a data scientist. Read my article: ‘6 Proven Steps To Becoming a Data Scientist [Complete Guide] for in-depth findings and recommendations! – This is perhaps the most comprehensive article on the subject you will find on the internet!
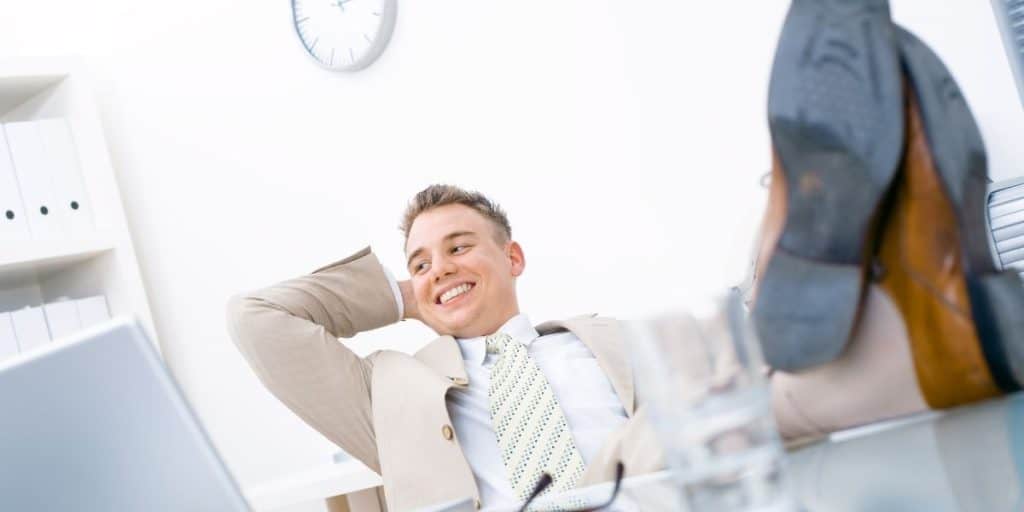
Table of Contents
What Is Data Science?
Data science is a newer field created by a combination of several classic disciplines. To be a successful data scientist, a student must have an excellent understanding of each. Then you will be able to predict results by analyzing data with the techniques below.
Statistics
You may associate statistics with probabilities, but it is so much more than that. Simply put, statistics tests out two sets of collected data to hypothesize the relationship between them. Usually, statisticians base their findings on a sample of the desired population, using the data to predict an outcome.
Data Mining
A company turns large masses of raw data into organized and useful information to develop new strategies to employ in the future. This use of data is called data mining. Data warehouse programs or databases store all the raw data in one place. Then the company uses mining software to search the data for patterns.
Databases
Companies store raw data in an organized way in databases. The ones with large quantities of data store them on physical database servers. The data is then easily accessible to use for multiple types of processes, many of which are related to data science.
Programming
There are different languages in programming, which is telling the computer to perform specific tasks. When you use coding to create a program, you enable automation, saving the user valuable time and money. Python and R are the most popular programming language used in data science.
Problem-Solving
Problem-solving is a process everyone learned in grade school. The same principles apply to solving more complex problems.
- What is the problem that needs solving and what are your concrete facts?
- Brainstorm ideas to solve the core problem.
- Choose the best idea to research and test out.
- Test out the selected theory and record any feedback and findings.
Machine Learning
Machine learning is not a classic discipline. It is relatively new compared to the others, but it is worth mentioning. It is the study of developing algorithms that enable computers to improve upon themselves based on experience. Machine learning branched out from the study of Artificial Intelligence (AI).
Qualities Found in a Successful Data Scientist
As previously mentioned, there are traits that a data scientist must possess to be successful in this field, as well as be happy on this career path.
Personality Traits
- A data scientist must be able to think with logic and clarity. The end goal must always be clear. Each undertaking must have a how and a why behind it.
- Being curious is what drives a data scientist to learn new things and to understand them fully. There is no growth and development without asking questions. The what-if and the why-not queries are part of what makes this field exciting.
- Being skeptical is related to being curious, but it is more in line with playing the devil’s advocate. Skepticism helps to balance out the effects of curiosity. Too much of one is not ideal.
- Seeing the big picture always helps with getting through the less exciting parts of data science. Understanding that the more menial tasks still contribute to the end goal is key to minimizing boredom and frustration with the job.
Important Skills
- A natural aptitude for analytics is essential. You can learn the process of analyzing data, but to have it come easy to you makes the work more enjoyable. A large portion of being a data analyst is spinning data to make it useful.
- Creative problem-solving skills come in handy when brainstorming solutions to problems. Thinking outside the box and being logical at the same time is an instrumental skill to have. A balance between inventive and linear thinking makes for a successful data scientist.
- Storytelling is a surprisingly useful skill for a data scientist to have. Not everyone on the team is an analyst. Therefore the ability to explain how the data fits into the big picture in colloquial terms is crucial.
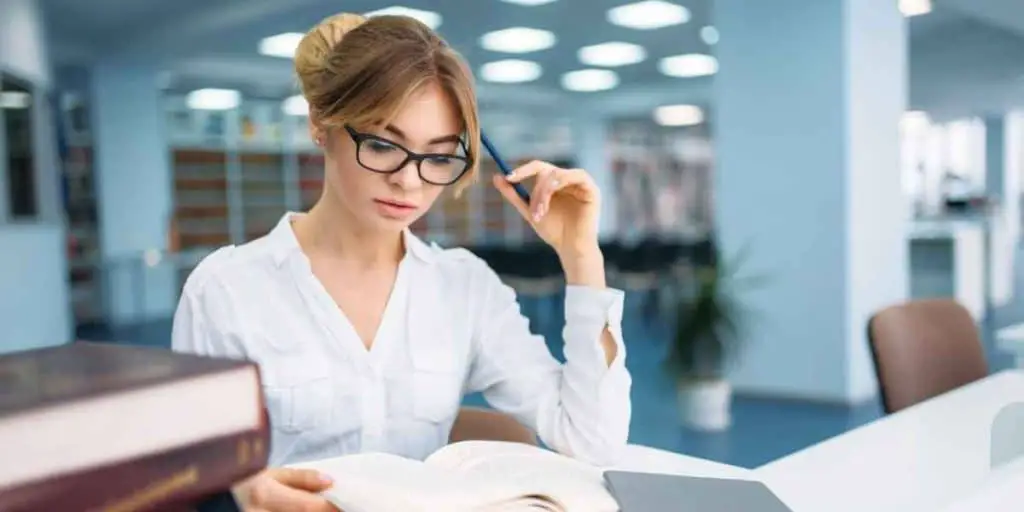
Tips and Tricks for Learning Data Science
- Hands-on learning. When looking for a school or program to earn your certifications, be sure there is sufficient opportunity built-in to the program for hands-on learning. Spending time on theories and satisfying your thirst for knowledge cannot replace what you learn by doing. Focus on the grander scheme of things.
- Understand how programming fits into the big picture. Learning to code with a programming language like Python is essential, but writing all codes from scratch is not. In the big picture, knowing when and how to use the code in data science is critical. Don’t write code from scratch for the sole purpose of proving that you can.
- Familiarize yourself with machine learning algorithms. As previously mentioned, machine learning is the cutting edge in data science. Be sure to familiarize yourself with machine learning and anything else new that comes along. Data science is always changing, so the top data scientists need to be as well.
- Don’t skip ahead. If you want to surge ahead and skip properly learning the foundations of data sciences, you might stumble and fall. There are programs out there that are self-paced. If you’re driven and work hard, you could receive your certifications in approximately six months.
7 Top-Rated Books on Popular Data Science Tools
- Hadoop: The Definitive Guide: Storage and Analysis at Internet Scale (Rated 4.5 out of 5 stars). This 4th edition guide will help you understand how to analyze datasets with Apache Hadoop.
- SAS Essentials: Mastering SAS for Data Analytics (Rated 4.6 out of 5 stars). This 2nd edition step-by-step text will help you explore the basics of using SAS to interpret and analyze data.
- Practical Tableau: 100 Tips, Tutorials, and Strategies from a Tableau Zen Master (Rated 4.5 out of 5 stars). This Tableau software manual will take you from the basics to more advanced concepts by finding useful data and showing it off visually.
- Hands-On Machine Learning with Scikit-Learn, Keras, & TensorFlow (Rated 4.8 out of 5 stars). This book will help you learn more about machine learning, including examples you can use to practice what you’ve learned.
- Spark: The Definitive Guide: Big Data Processing Made Simple (Rated 4.5 out of 5 stars). This guide will help you learn about how to use Apache Spark, as well as what is new in Spark 2.0.
- Data Mining for the Masses: w/ Implementations in RapidMiner & R (Rated 4.8 out of 5 stars). This 3rd edition text will teach you more about using RapidMiner and R with big data.
- Learn Excel 365 Expert Skills w/ The Smart Method (Rated 4.7 out of 5 stars). This 4th edition guide is the latest guide to accompany the July 2020 version of Excel 365.
Author’s Recommendations: Top Data Science Resources To Consider
Before concluding this article, I wanted to share few top data science resources that I have personally vetted for you. I am confident that you can greatly benefit in your data science journey by considering one or more of these resources.
- DataCamp: If you are a beginner focused towards building the foundational skills in data science, there is no better platform than DataCamp. Under one membership umbrella, DataCamp gives you access to 335+ data science courses. There is absolutely no other platform that comes anywhere close to this. Hence, if building foundational data science skills is your goal: Click Here to Sign Up For DataCamp Today!
- IBM Data Science Professional Certificate: If you are looking for a data science credential that has strong industry recognition but does not involve too heavy of an effort: Click Here To Enroll Into The IBM Data Science Professional Certificate Program Today! (To learn more: Check out my full review of this certificate program here)
- MITx MicroMasters Program in Data Science: If you are at a more advanced stage in your data science journey and looking to take your skills to the next level, there is no Non-Degree program better than MIT MicroMasters. Click Here To Enroll Into The MIT MicroMasters Program Today! (To learn more: Check out my full review of the MIT MicroMasters program here)
- Roadmap To Becoming a Data Scientist: If you have decided to become a data science professional but not fully sure how to get started: read my article – 6 Proven Ways To Becoming a Data Scientist. In this article, I share my findings from interviewing 100+ data science professionals at top companies (including – Google, Meta, Amazon, etc.) and give you a full roadmap to becoming a data scientist.
Conclusion
Data science is not easy to learn due to the number of disciplines a data scientist needs to master. It also takes a unique individual who is both logical and creative and curious and skeptical to be a successful data scientist. Taking the time to be trained the right way on the classic disciplines of data science will ensure that you build a strong foundation for a successful career.
BEFORE YOU GO: Don’t forget to check out my latest article – 6 Proven Steps To Becoming a Data Scientist [Complete Guide]. We interviewed numerous data science professionals (data scientists, hiring managers, recruiters – you name it) and created this comprehensive guide to help you land that perfect data science job.
Affiliate Disclosure: We participate in several affiliate programs and may be compensated if you make a purchase using our referral link, at no additional cost to you. You can, however, trust the integrity of our recommendation. Affiliate programs exist even for products that we are not recommending. We only choose to recommend you the products that we actually believe in.
Recent Posts
Data science has been a buzzword in recent years, and with the rapid advancements in artificial intelligence (AI) technologies, many wonder if data science as a field will be replaced by AI. As you...
In the world of technology, there's always something new and exciting grabbing our attention. Data science and analytics, in particular, have exploded onto the scene, with many professionals flocking...