There are many platforms for data analysis ranging from spreadsheet software to advanced statistical packages. These platforms have different levels of complexity; researchers choose the ideal platform based on their needs. So, is Excel adequate for data analysis?
Excel is not enough for data analysis. Excel spreadsheets may only be enough if you intend to run simple tasks, such as – percentages and distributions. However, Excel would not be adequate if you intend to go beyond the basics. For advanced analysis, Excel may be a bit risky to trust.
The rest of this article will focus on using excel for data analysis, when it is ideal to use excel, inputting data into Excel, limitations of Excel, and the right way to use it.
Important Sidenote: We interviewed numerous data science professionals (data scientists, hiring managers, recruiters – you name it) and identified 6 proven steps to follow for becoming a data scientist. Read my article: ‘6 Proven Steps To Becoming a Data Scientist [Complete Guide] for in-depth findings and recommendations! – This is perhaps the most comprehensive article on the subject you will find on the internet!
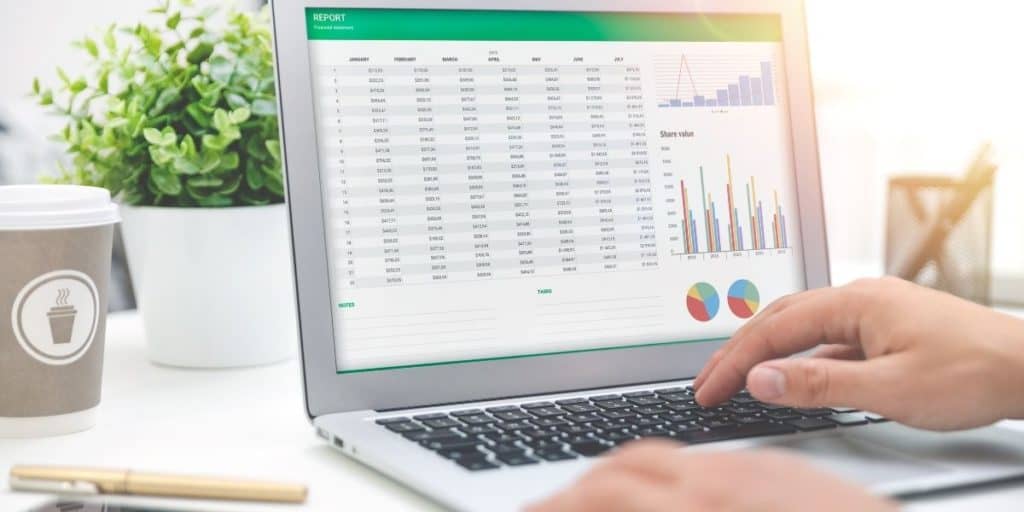
Table of Contents
Using Excel for Data Analysis
Microsoft Excel is one of the most popular tools for data analysis with built-in pivot tables. The program is not just ideal for basic data computations but also comes in handy in data analysis.
Excel offers unmatched convenience in data entry and allows researchers to quickly manipulate rows and columns before statistical analysis. The ability to analyze data is a powerful skill that enables people and organizations to make informed decisions.
When analyzing data using Excel, you have to start by selecting the cells that contain the data you intend to analyze. Then, press CTRL+Q or the quick analysis image button lies at the bottom right of the highlighted data. You can use the Excel formulas to aggregate data and create meaningful reports.
Here is a video to help you understand how to analyze data using Excel:
An excel spreadsheet organizes data into a readable format making it easy to extract actionable insights. If you are analyzing complex data, you can customize fields and actions. Compared to other software, Excel has easy to use charting functionality and other crucial built-in features. Most people find Excel ideal due to its ability to cut and paste data and charts into reports.
When Is It Ideal to Use Excel?
For a vast market research community, Excel is enough because they only deal with basic distribution statistics. Excel provides excellent graphics that researchers often use to spruce up their reports. Excel may be ideal if you seek to create decent looking charts, something that might be hard to achieve with SPSS. However, even if Excel exceeds SPSS in the graphical field, SPSS surpasses Excel in analytical capacity.
- What is good about Excel spreadsheets in data analysis? They are great tools for organizing data fast and efficiently. For instance, the vertical lookup capacity and the sorting functions in Excel are exceptional. Excel spreadsheets will serve you perfectly while analyzing the basic characteristics of your data. For example, if you want a simple report on the customers who are happy with your services, Excel analysis is enough.
- How far can you go with Excel for data analysis? You can use Excel to calculate correlations and t-tests to describe the relationships that exist within your data. However, beyond this analysis, it might be risky to rely on the Excel analysis results. Excel calculations can perform regression analysis alongside other forecasting analysis. Excel algorithms performing this analysis are not as advanced as other platforms.
Inputting Data Into Excel
There are several ways of getting data into Excel. The best way is to upload the data from a reliable source, while the worst is to copy and paste the data. Copying and pasting data into Excel open rooms for errors. You may alter the data without even realizing it. If you input the wrong figures into the spreadsheet, your entire data analysis will be useless.
You can pull or upload data into an Excel spreadsheet from many different sources. You could source the data from databases like Oracle, Db2, or Microsoft SQL Server.
You may also input data from applications like customer relationship management (CRM) or enterprise resource planning (ERP). If you are analyzing data from multiple sources, you should be able to consolidate it to ensure the results are in sync.
You should ensure that data is valid before inputting it into Excel for analysis. It would be demotivating to go too far into the data analysis to ensure that the data is not reliable.
To ensure that you only input valid data into the spreadsheets, you should consider the completeness of the data. Consider the age of the data to ensure that it is not outdated. You should also be sure that the data suits the context of your analysis.
Limitations of Excel for Data Analysis
Most researchers still use Excel for data analysis, and there is nothing wrong with that. After all, Excel provides an excellent way of collecting and analyzing small amounts of data. However, the limitations of Excel are evident when analysis requires large amounts of data. Researchers often experience several issues when performing complex data analysis with Excel.
Excel spreadsheets are prone to error because they have no error control. You may use the program for a long time only to realize that a macro was wrong by one cell. You then have to go back and determine when the error occurred, and this is often time-consuming. The program does not offer a testing frame or a debugging tool that researchers can use to determine if all cells are working as expected.
Researchers also encounter problematic scalability while using Excel to analyze data, mainly when research involves large data amounts. Researchers also report the speed of iteration of analysis as an issue of using Excel. Crashes and performance degradations when handling big data sets limit the professional use of Excel in data analysis. The row limit on Excel can be an issue while handling big data sets.
Excel is not made for collaboration in big teams. Most data engineers and data scientists do not work alone anymore. Most data analysts are a part of small or big labs that require constant communications on the applications they are working on.
Documentations, feature discussions, and team debugging are all important aspects while analyzing data. Manual modifications in Excel spreadsheets are often lost, making it to reproduce data.
The Excel algorithms are not as advanced as the ones you would find in other data analysis platforms like STATA, SAS, and SPSS. Though you can perform regression modeling in Excel, statistical software offers more than modeling. Statistical software allows analysts to make assumptions that improve the level of accuracy and indicate significance in a manner that Excel can’t.
Research indicates that 90% of spreadsheets contain an error. The preponderance of these errors increases as the calculations and analysis become more complex. The worrying part is that most of these errors go unnoticed, which could compromise Excel analysis results. By relying solely on Excel spreadsheets, you could be making decisions based on inaccurate and faulty data.
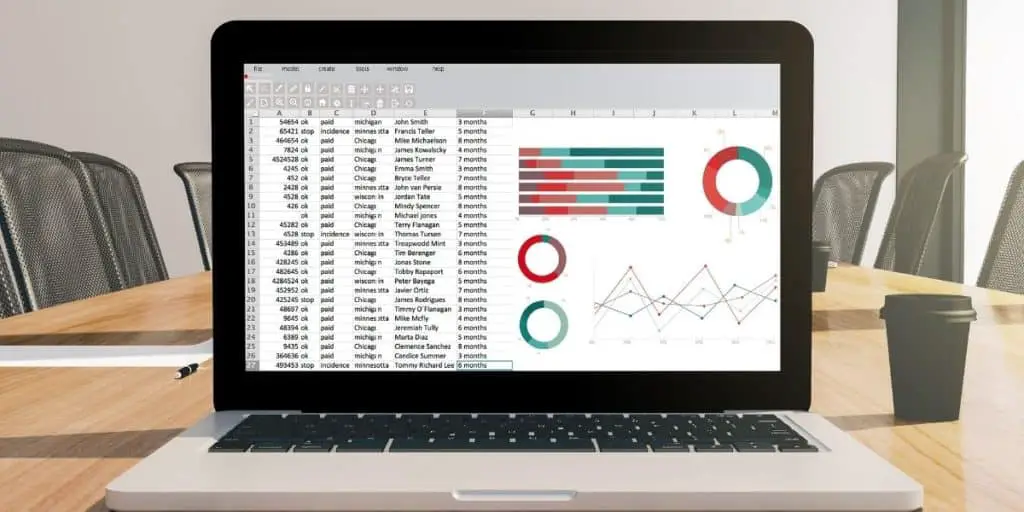
Using Excel the Right Way
Excel could add immense value to your organization if you learn the right way to use it. However, you should not rest all your data analysis expectations on Excel alone. Instead, you should use Excel alongside other applications; data analysis software makes up for Excel’s limitations. The best way to use Excel is to treat it as one of the many tools of data analysis in your organization.
Author’s Recommendations: Top Data Science Resources To Consider
Before concluding this article, I wanted to share few top data science resources that I have personally vetted for you. I am confident that you can greatly benefit in your data science journey by considering one or more of these resources.
- DataCamp: If you are a beginner focused towards building the foundational skills in data science, there is no better platform than DataCamp. Under one membership umbrella, DataCamp gives you access to 335+ data science courses. There is absolutely no other platform that comes anywhere close to this. Hence, if building foundational data science skills is your goal: Click Here to Sign Up For DataCamp Today!
- IBM Data Science Professional Certificate: If you are looking for a data science credential that has strong industry recognition but does not involve too heavy of an effort: Click Here To Enroll Into The IBM Data Science Professional Certificate Program Today! (To learn more: Check out my full review of this certificate program here)
- MITx MicroMasters Program in Data Science: If you are at a more advanced stage in your data science journey and looking to take your skills to the next level, there is no Non-Degree program better than MIT MicroMasters. Click Here To Enroll Into The MIT MicroMasters Program Today! (To learn more: Check out my full review of the MIT MicroMasters program here)
- Roadmap To Becoming a Data Scientist: If you have decided to become a data science professional but not fully sure how to get started: read my article – 6 Proven Ways To Becoming a Data Scientist. In this article, I share my findings from interviewing 100+ data science professionals at top companies (including – Google, Meta, Amazon, etc.) and give you a full roadmap to becoming a data scientist.
Conclusion
Compared to statistical packages, Excel is easier to learn and use. Programs like SPSS and SAS take knowledge and training to utilize their full knowledge.
However, if research calls for deeper analysis than the basic, the statistical packages will deliver more illustrative and solid results. It is advisable to use a combination of different data analysis programs, including Excel spreadsheets. Understanding the functions and limitations of each is crucial.
BEFORE YOU GO: Don’t forget to check out my latest article – 6 Proven Steps To Becoming a Data Scientist [Complete Guide]. We interviewed numerous data science professionals (data scientists, hiring managers, recruiters – you name it) and created this comprehensive guide to help you land that perfect data science job.
Affiliate Disclosure: We participate in several affiliate programs and may be compensated if you make a purchase using our referral link, at no additional cost to you. You can, however, trust the integrity of our recommendation. Affiliate programs exist even for products that we are not recommending. We only choose to recommend you the products that we actually believe in.
Recent Posts
Data science has been a buzzword in recent years, and with the rapid advancements in artificial intelligence (AI) technologies, many wonder if data science as a field will be replaced by AI. As you...
In the world of technology, there's always something new and exciting grabbing our attention. Data science and analytics, in particular, have exploded onto the scene, with many professionals flocking...