If you are involved in statistical computing or data analysis, you will likely be familiar with the SQL and R computing languages. At some point, it would be natural to ask: which language is harder to learn and use?
SQL is not harder than R in terms of complexity of usage and ease of learning. SQL is a domain-specific language and has been established as a standard by multiple standardization organizations. It makes the theoretical understanding and practical application of SQL simpler for all users.
This article will cover the key areas that make SQL stand out as being easier over the R language. It will also highlight areas where it is not equitable to compare both languages. If you are currently debating whether you should learn one or both languages, read on and discover what option is best for you.
Important Sidenote: We interviewed numerous data science professionals (data scientists, hiring managers, recruiters – you name it) and identified 6 proven steps to follow for becoming a data scientist. Read my article: ‘6 Proven Steps To Becoming a Data Scientist [Complete Guide] for in-depth findings and recommendations! – This is perhaps the most comprehensive article on the subject you will find on the internet!
Table of Contents
Difference Between Data Querying and Data Analysis
To properly compare SQL to R in terms of difficulty, it is essential to understand the differences between data querying and data analysis fundamentals.
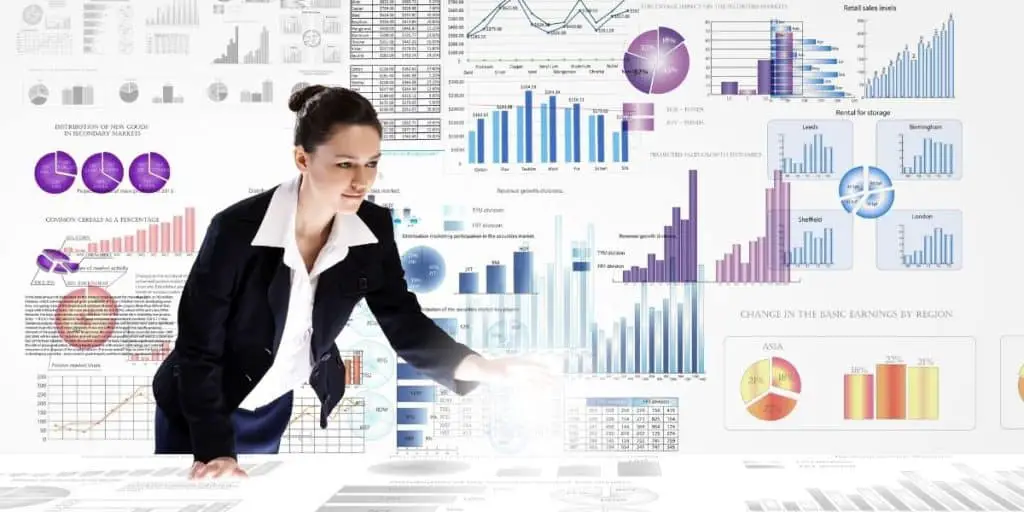
The former involves searching through volumes of data finding relationships between individual entries and variables. The latter consists of applying a set of calculations on retrieved data collections to search for different patterns. Data analysis examines unique sets of data to extract useful information.
This distinction is important when comparing SQL to R. This is because SQL excels at querying large amounts of structured data but can encounter efficiency issues when attempting to perform complex analytical calculations on the data that it has retrieved.
On the other hand, R can be more efficient when statistical analysis needs to be applied or to create visualizations of the analysis that is to be reported.
Different Focal Roles of SQL and R
SQL is a domain-specific language. It was developed to query relational databases. It has been designed to access multiple records using as few as a single command. Additionally, you can access these records with or without an index being present.
As a result, SQL is very efficient in querying large volumes of data across multiple tables dispersed over various databases.
As a declarative language, the syntax used in SQL commands is relatively easy to learn compared to other programming languages.
The R language is not domain-specific—it is a general-purpose programming language. Its focus, however, is on statistical computing and graphics. Theoretically, you could use the R language to conduct queries on large databases. However, there is a substantial practical limitation to the R language that SQL does not experience.
When using R, it runs entirely on RAM. SQL runs on the database server or the collection of machines that comprise the database cluster.
SQL requires approximately 15 to 20 percent of RAM compared to the size of the data set that it is querying to remain efficient in terms of response time and latency. R, on the other hand, works entirely on RAM. It can create processing issues when the analytical or statistical calculus to be run is unusually complex.
The Intersection Between SQL and R
The key intersection between both languages resides with data. More specifically, how data is queried, aggregated, parsed, and analyzed.
When the comparison between both languages is viewed at this point of intersection, the question of difficulty and complexity can be divorced from how easy or difficult they are to learn and transitioned to ease of use in specific scenarios.
For example, for a simple single command query to be run on an extensive relational database, SQL would be the preferred choice. On the other hand, attempting to perform a complex set of data transformations utilizing simple syntax would be more complicated when attempted with SQL.
Combining aggregate and non-aggregate calculations in the way that R allows, makes achieving your operation possible with a more straightforward process. Attempting the same results with SQL would require more complex data manipulation and added processing power.
Pivoting from wide datasets to long datasets requires writing very complex query code with SQL. With R, doing so can be done with a minimal amount of code.
It is easier to run predictions, modeling, and clustering with R. The plotting and charting functionality offered by R offers a wealth of flexibility and customization that permits you a more comprehensive range of plot creation than that found on platforms such as Tableau and Google Data Studio.
Distinguishing Practicality Over Ease of Learnability
Considering what we have covered so far, it should become more apparent how making blanket statements about how one language is more challenging than another is not always fair.
For example, there is no denying that the learning curve for R is steep compared to SQL. However, part of the reason this is so is that there is a degree of modularity in learning SQL. You can learn it in stages. Such a luxury does not come with R because it is not a domain-specific language.
As with other general-purpose programming languages, R’s range of functionality implies an extended learning cycle compared to SQL.
Such an extended learning process can be seen as a burden, especially if your need for the benefits of R is occasional. However, if you plan to be involved heavily with complex analysis, statistics, predictions, and modeling, the dividends of such an investment in time and effort can be worthwhile.
Using R Without Learning R
Likewise, if your involvement with data will be limited to simple queries and basic data analysis, taking the time to learn R might be a bit of an overkill.
There are business analytics services, such as Microsoft’s Power BI, that offer R-powered visualization integration with external data sources within a user-friendly interface for those who are only seeking R’s visualization benefits. Such services provide you with the visualization creation capability of R without the burden of the steep learning curve generally associated with it.
Using SQL in Combination With R
Sometimes, the way to exact the most efficiency out of any language is to combine it with another. Data workflows, especially those involving complex calculations combined with the requirement for deep querying of large databases, can be well served by combining SQL with R.
In such situations, the workflows are structured to use SQL to gather the data required for more in-depth analysis into a single table. Subsequently, R is used to run the analysis scripts that are needed.
Once you have practical knowledge of R, you can speed up the coding process by relying on the many scripts found in R’s libraries. These scripts accommodate a wide range of in-depth analysis and statistical scenarios.
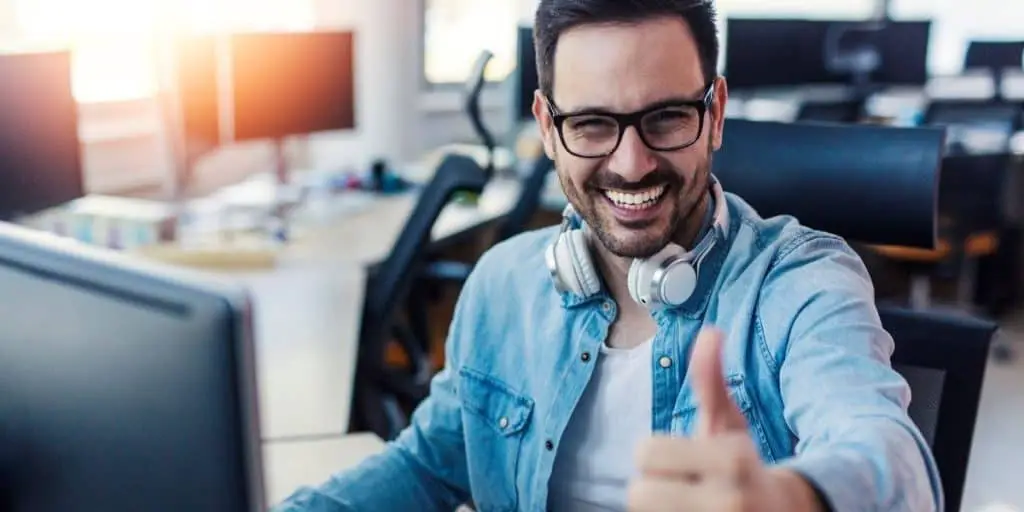
Sequential Intuitiveness
One more thing that merits mentioning R is what can be described as R’s sequential fomenting of user intuitiveness. Running a thorough analysis workflow using R does not involve a single process.
Typically, it involves running multiple steps sequentially. Sometimes, these steps can include data transformation and circling back to the first step of the process numerous times. While this might seem cumbersome, it offers a degree of interactivity and intimacy with the analyzed data, allowing for improved control and understanding of the output.
Author’s Recommendations: Top Data Science Resources To Consider
Before concluding this article, I wanted to share few top data science resources that I have personally vetted for you. I am confident that you can greatly benefit in your data science journey by considering one or more of these resources.
- DataCamp: If you are a beginner focused towards building the foundational skills in data science, there is no better platform than DataCamp. Under one membership umbrella, DataCamp gives you access to 335+ data science courses. There is absolutely no other platform that comes anywhere close to this. Hence, if building foundational data science skills is your goal: Click Here to Sign Up For DataCamp Today!
- IBM Data Science Professional Certificate: If you are looking for a data science credential that has strong industry recognition but does not involve too heavy of an effort: Click Here To Enroll Into The IBM Data Science Professional Certificate Program Today! (To learn more: Check out my full review of this certificate program here)
- MITx MicroMasters Program in Data Science: If you are at a more advanced stage in your data science journey and looking to take your skills to the next level, there is no Non-Degree program better than MIT MicroMasters. Click Here To Enroll Into The MIT MicroMasters Program Today! (To learn more: Check out my full review of the MIT MicroMasters program here)
- Roadmap To Becoming a Data Scientist: If you have decided to become a data science professional but not fully sure how to get started: read my article – 6 Proven Ways To Becoming a Data Scientist. In this article, I share my findings from interviewing 100+ data science professionals at top companies (including – Google, Meta, Amazon, etc.) and give you a full roadmap to becoming a data scientist.
Conclusion
Based strictly on learnability, SQL is easier to learn than R. When measured against each other in terms of usability, R emerges as more complex. Such complexity can be a hindrance to those whose work with data does not require it.
In short, SQL is easier to learn and easier to use when the primary purpose involves querying structured data in a relational database. However, when such data has already been collected into a single table and needs sequential processing for statistical study, R can make those processes simpler to manage.
BEFORE YOU GO: Don’t forget to check out my latest article – 6 Proven Steps To Becoming a Data Scientist [Complete Guide]. We interviewed numerous data science professionals (data scientists, hiring managers, recruiters – you name it) and created this comprehensive guide to help you land that perfect data science job.
Affiliate Disclosure: We participate in several affiliate programs and may be compensated if you make a purchase using our referral link, at no additional cost to you. You can, however, trust the integrity of our recommendation. Affiliate programs exist even for products that we are not recommending. We only choose to recommend you the products that we actually believe in.
Recent Posts
Data science has been a buzzword in recent years, and with the rapid advancements in artificial intelligence (AI) technologies, many wonder if data science as a field will be replaced by AI. As you...
In the world of technology, there's always something new and exciting grabbing our attention. Data science and analytics, in particular, have exploded onto the scene, with many professionals flocking...