In the world of statistics, you may have come across the terms p-value and critical value. These concepts are essential in hypothesis testing, a process that helps you make informed decisions based on data. As you embark on your journey to understand the significance and applications of these values, don’t worry; you’re not alone. Many professionals and students alike grapple with these concepts, but once you get the hang of what they mean, they become powerful tools at your fingertips.
The main difference between p-value and critical value is that the p-value quantifies the strength of evidence against a null hypothesis, while the critical value sets a threshold for assessing the significance of a test statistic. Simply put, if your p-value is below the critical value, you reject the null hypothesis.
As you read on, you can expect to dive deeper into the definitions, applications, and interpretations of these often misunderstood statistical concepts. The remainder of the article will guide you through how p-values and critical values work in real-world scenarios, tips on interpreting their results, and potential pitfalls to avoid. By the end, you’ll have a clear understanding of their role in hypothesis testing, helping you become a more effective researcher or analyst.
Important Sidenote: We interviewed numerous data science professionals (data scientists, hiring managers, recruiters – you name it) and identified 6 proven steps to follow for becoming a data scientist. Read my article: ‘6 Proven Steps To Becoming a Data Scientist [Complete Guide] for in-depth findings and recommendations! – This is perhaps the most comprehensive article on the subject you will find on the internet!
Table of Contents
Understanding P-Value and Critical Value
When you dive into the world of statistics, it’s essential to grasp the concepts of P-value and critical value. These two values play a crucial role in hypothesis testing, helping you make informed decisions based on data. In this section, we will focus on the concept of hypothesis testing and how P-value and critical value relate to it.
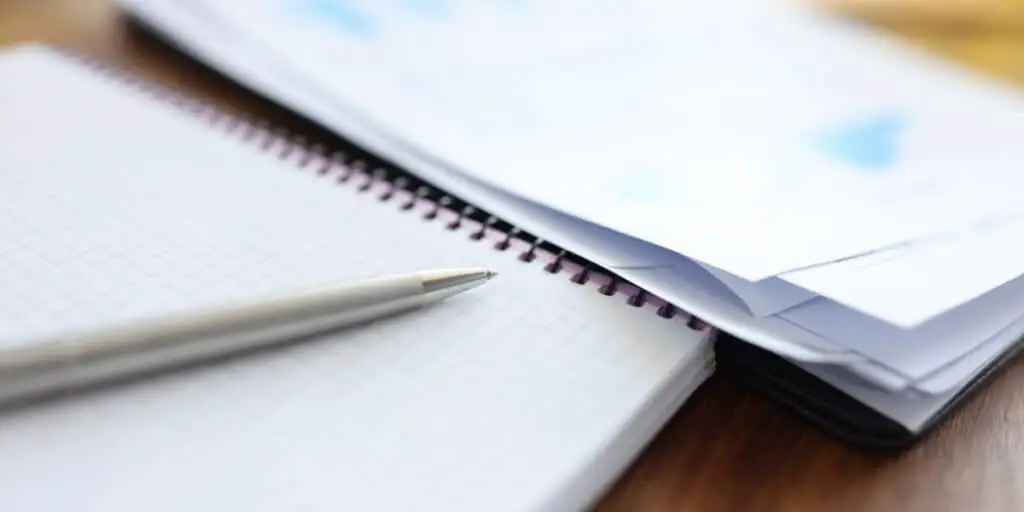
Concept of Hypothesis Testing
Hypothesis testing is a statistical technique used to analyze data and draw conclusions. You start by creating a null hypothesis (H0) and an alternative hypothesis (H1). The null hypothesis represents the idea that there is no significant effect or relationship between the variables being tested, while the alternative hypothesis claims that there is a significant effect or relationship.
To conduct a hypothesis test, follow these steps:
- Formulate your null and alternative hypotheses.
- Choose an appropriate statistical test and significance level (α).
- Collect and analyze your data.
- Calculate the test statistic and P-value.
- Compare the P-value to the critical value.
Now, let’s discuss how P-value and critical value come into play during hypothesis testing.
P-Value
The P-value is the probability of observing a test statistic as extreme (or more extreme) than the one calculated if the null hypothesis were true. In simpler terms, it’s the likelihood of getting your observed results by chance alone. The lower the P-value, the more evidence you have against the null hypothesis.
Here’s what you need to know about P-values:
- A low P-value (typically ≤ 0.05) indicates that the null hypothesis is unlikely to be true.
- A high P-value (typically > 0.05) suggests that the observed results align with the null hypothesis.
Critical Value
The critical value is a threshold that defines whether the test statistic is extreme enough to reject the null hypothesis. It depends on the chosen significance level (α) and the specific statistical test being used. If the test statistic exceeds the critical value, you reject the null hypothesis in favor of the alternative.
To summarize:
- If the P-value ≤ critical value, reject the null hypothesis.
- If the P-value > critical value, fail to reject the null hypothesis (do not conclude that the alternative is true).
In conclusion, understanding P-value and critical value is crucial for hypothesis testing. They help you determine the significance of your findings and make data-driven decisions. By grasping these concepts, you’ll be well-equipped to analyze data and draw meaningful conclusions in a variety of contexts.
P-Value Essentials
Calculating and interpreting p-values is essential to understanding statistical significance in research. In this section, we’ll cover the basics of p-values and how they relate to critical values.
Calculating P-Values
A p-value represents the probability of obtaining a result at least as extreme as the observed data, assuming the null hypothesis is correct. To calculate a p-value, follow these steps:
- Define your null and alternative hypotheses.
- Determine the test statistic and its distribution.
- Calculate the observed test statistic based on your sample data.
- Find the probability of obtaining a test statistic at least as extreme as the observed value.
Let’s dive deeper into these steps:
- Step 1: Formulate the null hypothesis (H₀) and alternative hypothesis (H₁). The null hypothesis typically states that there is no effect or relationship between variables, while the alternative hypothesis suggests otherwise.
- Step 2: Determine your test statistic and its distribution. The choice of test statistic depends on your data and hypotheses. Some common test statistics include the t-test, z-test, or chi-square test.
- Step 3: Using your sample data, compute the test statistic. This value quantifies the difference between your sample data and the null hypothesis.
- Step 4: Find the probability of obtaining a test statistic at least as extreme as the observed value, under the assumption that the null hypothesis is true. This probability is the p-value.
Interpreting P-Values
Once you’ve calculated the p-value, it’s time to interpret your results. The interpretation depends on the pre-specified significance level (α) you’ve chosen. Here’s a simplified guideline:
- If p-value ≤ α, you can reject the null hypothesis.
- If p-value > α, you cannot reject the null hypothesis.
Keep in mind that:
- A lower p-value indicates stronger evidence against the null hypothesis.
- A higher p-value implies weaker evidence against the null hypothesis.
Remember that statistical significance (p-value ≤ α) does not guarantee practical or scientific significance. It’s essential not to take the p-value as the sole metric for decision-making, but rather as a tool to help gauge your research outcomes.
In summary, p-values are crucial in understanding and interpreting statistical research results. By calculating and appropriately interpreting p-values, you can deepen your knowledge of your data and make informed decisions based on statistical evidence.
Critical Value Essentials
In this section, we’ll discuss two important aspects of critical values: Significance Level and Rejection Region. Knowing these concepts helps you better understand hypothesis testing and make informed decisions about the statistical significance of your results.
Significance Level
The significance level, often denoted as α or alpha, is an essential part of hypothesis testing. You can think of it as the threshold for deciding whether your results are statistically significant or not. In general, a common significance level is 0.05 or 5%, which means that there is a 5% chance of rejecting a true null hypothesis.
To help you understand better, here are a few key points:
- The lower the significance level, the more stringent the test.
- Higher α-levels may increase the risk of Type I errors (incorrectly rejecting the null hypothesis).
- Lower α-levels may increase the risk of Type II errors (failing to reject a false null hypothesis).
Rejection Region
The rejection region is the range of values that, if your test statistic falls within, leads to the rejection of the null hypothesis. This area depends on the critical value and the significance level. The critical value is a specific point that separates the rejection region from the rest of the distribution. Test statistics that fall in the rejection region provide evidence that the null hypothesis might not be true and should be rejected.
Here are essential points to consider when using the rejection region:
- Z-score: The z-score is a measure of how many standard deviations away from the mean a given value is. If your test statistic lies in the rejection region, it means that the z-score is significant.
- Rejection regions are tailored for both one-tailed and two-tailed tests.
- In a one-tailed test, the rejection region is either on the left or right side of the distribution.
- In a two-tailed test, there are two rejection regions, one on each side of the distribution.
By understanding and considering the significance level and rejection region, you can more effectively interpret your statistical results and avoid making false assumptions or claims. Remember that critical values are crucial in determining whether to reject or accept the null hypothesis.
Statistical Tests and Decision Making
T-Test
When you’re comparing the means of two samples, a t-test is often used. This test helps you determine whether there is a significant difference between the means. Here’s how you can conduct a t-test:
- Calculate the t-statistic for your samples
- Determine the degrees of freedom
- Compare the t-statistic to a critical value from a t-distribution table
If the t-statistic is greater than the critical value, you can reject the null hypothesis and conclude that there is a significant difference between the sample means. Some key points about t-test:
- Test statistic: In a t-test, the t-statistic is the key value that you calculate
- Sample: For a t-test, you’ll need two independent samples to compare
ANOVA
The Analysis of Variance (ANOVA) is another statistical test, often used when you want to compare the means of three or more treatment groups. With this method, you analyze the differences between group means and make decisions on whether the total variation in the dataset can be accounted for by the variance within the groups or the variance between the groups. Here are the main steps in conducting an ANOVA test:
- Calculate the F statistic
- Determine the degrees of freedom for between-groups and within-groups
- Compare the F statistic to a critical value from an F-distribution table
When the F statistic is larger than the critical value, you can reject the null hypothesis and conclude that there is a significant difference among the treatment groups. Keep these points in mind for ANOVA tests:
- Treatment Groups: ANOVA tests require three or more groups to compare
- Observations: You need multiple observations within each treatment group
Confidence Intervals
Confidence intervals (CIs) are a way to estimate values within a certain range, with a specified level of confidence. They help to indicate the reliability of an estimated parameter, like the mean or difference between sample means. Here’s what you need to know about calculating confidence intervals:
- Determine the point estimate (e.g., sample mean or difference in means)
- Calculate the standard error
- Multiply the standard error by the appropriate critical value
The result gives you a range within which the true population parameter is likely to fall, with a certain level of confidence (e.g., 95%). Remember these insights when working with confidence intervals:
- Confidence Level: The confidence level is the probability that the true population parameter falls within the calculated interval
- Critical Value: Based on the specified confidence level, you’ll determine a critical value from a table (e.g., t-distribution)
Remember, using appropriate statistical tests, test statistics, and critical values will help you make informed decisions in your data analysis.
Comparing P-Values and Critical Values
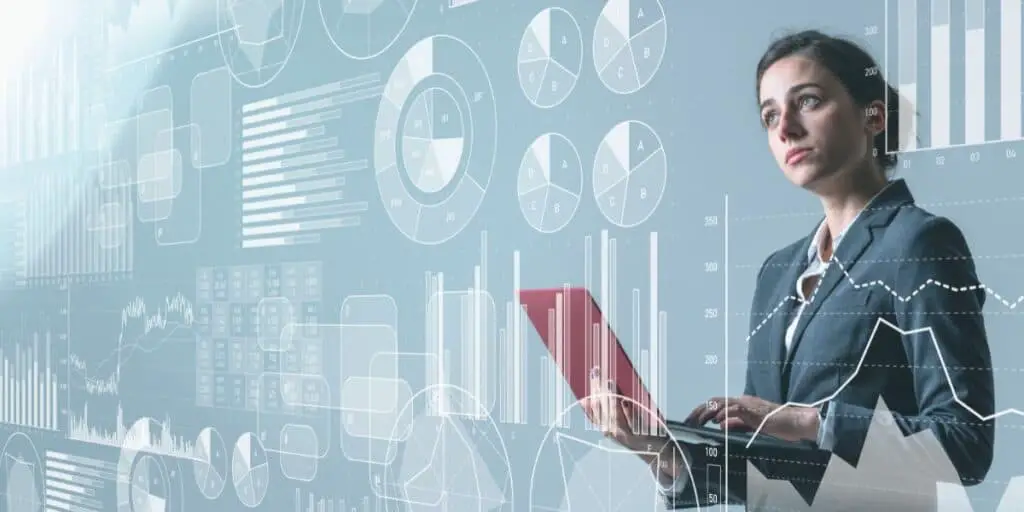
Differences and Similarities
When analyzing data, you may come across two important concepts – p-values and critical values. While they both help determine the significance of a data set, they have some differences and similarities.
- P-values are probabilities, ranging from 0 to 1, indicating how likely it is a particular result could be observed if the null hypothesis is true. Lower p-values suggest the null hypothesis should be rejected, meaning the observed data is not due to chance alone.
- On the other hand, critical values are preset thresholds that decide whether the null hypothesis should be rejected or not. Results that surpass the critical value support adopting the alternative hypothesis.
The main similarity between p-values and critical values is their role in hypothesis testing. Both are used to determine if observed data provides enough evidence to reject the null hypothesis in favor of the alternative hypothesis.
Applications in Geospatial Data Analysis
In the field of geospatial data analysis, p-values and critical values play essential roles in making data-driven decisions. Researchers like Hartmann, Krois, and Waske from the Department of Earth Sciences at Freie Universitaet Berlin often use these concepts in their e-Learning project SOGA.
To better understand the applications, let’s look at three main aspects:
- Spatial autocorrelation: With geospatial data, points might be related not only by their values but also by their locations. P-values can help assess spatial autocorrelation and recognize underlying spatial patterns.
- Geostatistical analysis: Techniques like kriging or semivariogram estimation depend on critical values and p-values to decide the suitability of a model. By finding the best fit model, geospatial data can be better represented, ensuring accurate and precise predictions.
- Comparing geospatial data groups: When comparing two subsets of data (e.g., mineral concentrations, soil types), p-values can be used in permutation tests or t-tests to verify if the observed differences are significant or due to chance.
In summary, when working with geospatial data analysis, p-values and critical values are crucial tools that enable you to make informed decisions about your data and its implications. By understanding the differences and similarities between the two concepts, you can apply them effectively in your geospatial data analysis journey.
Standard Distributions and Scores
In this section, we will discuss the Standard Normal Distribution and its associated scores, namely Z-Score and T-Statistic. These concepts are crucial in understanding the differences between p-values and critical values.
Standard Normal Distribution
The Standard Normal Distribution is a probability distribution that has a mean of 0 and a standard deviation of 1. This distribution is crucial for hypothesis testing, as it helps you make inferences about your data based on standard deviations from the mean. Some characteristics of this distribution include:
- 68% of the data falls within ±1 standard deviation from the mean
- 95% of the data falls within ±2 standard deviations from the mean
- 99.7% of the data falls within ±3 standard deviations from the mean
Z-Score
The Z-Score is a measure of how many standard deviations away a data point is from the mean of the distribution. It is used to compare data points across different distributions with different means and standard deviations. To calculate the Z-Score, use the formula:
Z-Score = (Value - Mean) / Standard Deviation
Key features of the Z-Score include:
- Positive Z-Scores indicate values above the mean
- Negative Z-Scores indicate values below the mean
- A Z-Score of 0 is equal to the mean
T-Statistic
The T-Statistic, also known as the Student’s t-distribution, is another way to assess how far away a data point is from the mean. It comes in handy when:
- You have a small sample size (generally less than 30)
- Population variance is not known
- Population is assumed to be normally distributed
The T-Statistic shares similarities with the Z-Score but adjusts for sample size, making it more appropriate for smaller samples. The formula for calculating the T-Statistic is:
T-Statistic = (Sample Mean - Population Mean) / (Sample Standard Deviation / √Sample Size)
In conclusion, understanding the Standard Normal Distribution, Z-Score, and T-Statistic will help you better differentiate between p-values and critical values, ultimately aiding in accurate statistical analysis and hypothesis testing.
Author’s Recommendations: Top Data Science Resources To Consider
Before concluding this article, I wanted to share few top data science resources that I have personally vetted for you. I am confident that you can greatly benefit in your data science journey by considering one or more of these resources.
- DataCamp: If you are a beginner focused towards building the foundational skills in data science, there is no better platform than DataCamp. Under one membership umbrella, DataCamp gives you access to 335+ data science courses. There is absolutely no other platform that comes anywhere close to this. Hence, if building foundational data science skills is your goal: Click Here to Sign Up For DataCamp Today!
- IBM Data Science Professional Certificate: If you are looking for a data science credential that has strong industry recognition but does not involve too heavy of an effort: Click Here To Enroll Into The IBM Data Science Professional Certificate Program Today! (To learn more: Check out my full review of this certificate program here)
- MITx MicroMasters Program in Data Science: If you are at a more advanced stage in your data science journey and looking to take your skills to the next level, there is no Non-Degree program better than MIT MicroMasters. Click Here To Enroll Into The MIT MicroMasters Program Today! (To learn more: Check out my full review of the MIT MicroMasters program here)
- Roadmap To Becoming a Data Scientist: If you have decided to become a data science professional but not fully sure how to get started: read my article – 6 Proven Ways To Becoming a Data Scientist. In this article, I share my findings from interviewing 100+ data science professionals at top companies (including – Google, Meta, Amazon, etc.) and give you a full roadmap to becoming a data scientist.
Frequently Asked Questions
What is the relationship between p-value and critical value?
The p-value represents the probability of observing the test statistic under the null hypothesis, while the critical value is a predetermined threshold for declaring significance. If the p-value is less than the critical value, you reject the null hypothesis.
How do you interpret p-value in comparison to critical value?
When the p-value is smaller than the critical value, there is strong evidence against the null hypothesis, which means you reject it. In contrast, if the p-value is larger, you fail to reject the null hypothesis and cannot conclude a significant effect.
What does it mean when the p-value is greater than the critical value?
If the p-value is greater than the critical value, it indicates that the observed data are consistent with the null hypothesis, and you do not have enough evidence to reject it. In other words, the finding is not statistically significant.
How are critical values used to determine significance?
Critical values are used as a threshold to determine if a test statistic is considered significant. When the test statistic is more extreme than the critical value, you reject the null hypothesis, indicating that the observed effect is unlikely due to chance alone.
Why is it important to know both p-value and critical value in hypothesis testing?
Knowing both p-value and critical value helps you to:
- Understand the strength of evidence against the null hypothesis
- Decide whether to reject or fail to reject the null hypothesis
- Assess the statistical significance of your findings
- Avoid misinterpretations and false conclusions
How do you calculate critical values and compare them to p-values?
To calculate critical values, you:
- Choose a significance level (α)
- Determine the appropriate test statistic distribution
- Find the value that corresponds to α in the distribution
Then, you compare the calculated critical value with the p-value to determine if the result is statistically significant or not. If the p-value is less than the critical value, you reject the null hypothesis.
BEFORE YOU GO: Don’t forget to check out my latest article – 6 Proven Steps To Becoming a Data Scientist [Complete Guide]. We interviewed numerous data science professionals (data scientists, hiring managers, recruiters – you name it) and created this comprehensive guide to help you land that perfect data science job.
Affiliate Disclosure: We participate in several affiliate programs and may be compensated if you make a purchase using our referral link, at no additional cost to you. You can, however, trust the integrity of our recommendation. Affiliate programs exist even for products that we are not recommending. We only choose to recommend you the products that we actually believe in.
Recent Posts
Data science has been a buzzword in recent years, and with the rapid advancements in artificial intelligence (AI) technologies, many wonder if data science as a field will be replaced by AI. As you...
In the world of technology, there's always something new and exciting grabbing our attention. Data science and analytics, in particular, have exploded onto the scene, with many professionals flocking...